Long-Term Health Monitoring Data Processing on Post-Tensioned Concrete Box-Girder Bridge by Wavelet-Based
Akhmad Aminullah, Department of Civil and Environmental Engineering, Universitas Gadjah Mada, Yogyakarta 55281, Indonesia
Iman Satyarno, Department of Civil and Environmental Engineering, Universitas Gadjah Mada, Yogyakarta 55281, Indonesia
Abstract
The concrete box-girder bridge is designed to have a long service life of around 100 years. To ensure safety and performance degradation during long service life, a Structural Health Monitoring System (SHMS) has been implemented in the box-girder bridge. SHMS can reliably assess structural response due to real-time applied loads, detect anomaly activities and locate the structural damage in the structure. Several sensors have been implemented in the bridge to continuously record the behavior of the bridge in all environmental conditions. Due to real-time natural conditions, false alarms occur frequently in SHM due to the disruption of noises and lead to misunderstanding of who is evaluating. Nevertheless, numerous SHM data that have been collected make it complicated to determine the anomaly of the structures. Therefore, it required signal processing to maximize the potentialities of the massive SHM data, as well as the efficiency of the time work. In this study, wavelet transformation, a rapid and unsupervised signal processing approach, was used to analyze the huge signal data by removing noise, and separating different signal sources as well. Further, with time-frequency analysis and multi-resolution capabilities, the transformation of wavelet is a promising tool for analyzing long-term SHM data. The suggested approach is shown by using long-term strain data from a 40 m concrete box-girder bridge in 24h. The results showed that after the denoising process, the highest discrepancy between the reconstructed and original strain signal is 2.73 μƐ and lost their energy less than 1%. Hence, the strain gauge sensor was successfully able to eliminate the noise through wavelet technology.
Keywords
Full Text:
PDFReferences
B. S. Widodo, “Aplikasi Wavelet Untuk Penghilangan Derau Isyarat Elektrokardiograf,” Jurnal Buana Informatika, Vol. %1 dari %2 Volume 2, Nomor 2, pp. 95-101, Juli 2011.
F. N. Catbas, “Structural health monitoring: applications and data analysis,” Woodhead, 2009.
Jinsuk Yim, Yinghong Cao, Ming L. Wang, “Monitoring a Post-Tensioned Concrete Box Girder Bridge with Cracks,” dalam SPIE Smart Structures and Materials & Nondestructive Evaluation and Health Monitoring, California, 2009.
Y. D. P. S. H. Z. a. F. G. Kang Yang, “Modeling of Temperature Time-Lag Efect for Concrete Box-Girder Bridges,” Applied Science, 2019.
M. M. Reda Taha, A. Noureldin, J. L. Lucero and T. J. Baca, “Wavelet Transform for Structural Health Monitoring: A Compendium of Uses and Features,” Sage, vol. Vol 5(3): 0267–29, 2006.
You-Liang Ding, Gao-XinWang, Peng Sun, Lai-Yi Wu, and Qing Yue, “Long-Term Structural Health Monitoring System for a High-Speed Railway Bridge Structure,” Hindawi, 2015.
J. Y. Y. Z. a. M. L. Yinghong Cao, “Temperature effects on cable stayed bridge using health monitoring system: a case study,” Structure Health Monitoring, 2010.
N. Y. Xia Y.X, “Wavelet-Based Signal Processing of Large SHM Data,” Asia-Pasific Workshop on Structural Health Monitoring, 2018.
Y.-X. Xia, “Integration of Long-Term SHM Data into Bridge Condition Assessment,” 2017.
M. L. Wang, “Mechanism of the effect of temperature on frequency based on long-term monitoring of an arch bridge,” US-Taiwan Workshop on Smart Structural Technology for Seismic Hazard Mitigation, 2004.
A. A. a. I. S. Rifdah R Z, “Analysis of the effect of temperature on the structure of box girder bridge using the long-term structural health monitoring system: wavelet transformation (case study: AP. Pettarani Makassar elevated toll bridge).,” In Press, 2022.
W.-X. a. D. R. G. Ren, “Structural damage identification using modal data. I: simulation verification.,” ASCE Journal of Structural Engineering, vol. 128(1) 87–95, 2002.
D. Donoho, “De-noising by soft-thresholding,” IEEE Transactions on Information , 1995.
J.-T. R. Y.-S. C. H.-M. a. S. N. Kim, “Damage identification in beam-type structures: requency-based method vs. mode-shape-based method,” Engineering Structures, pp. 25, 57–67., 2003.
Q. L. Q. J. H. W. J. C. a. Q. A. Xinghong Jiang, “An Improved Wavelet Threshold Denoising Method for Health Monitoring Data: A Case Study of the Hong Kong-Zhuhai-Macao Bridge Immersed Tunnel,” Applied Sciences, 2022.
F. Ningsih, Ekstraksi Ciri dan Identifikasi Sinyal Suara Jantung S1 Dan S2 Phonocardiogram (PCG) Menggunakan Metode Continuous Wavelet Transform, 2018.
L. Y. P. C. Y. W. Peiqiong Yu, "Wavelet De-noising with Improved Threshold Method for Bridge Health Monitoring," Vols. 978-1-4673-7687-7, 2015.
DOI: https://doi.org/10.21831/inersia.v19i1.54918
Refbacks
- There are currently no refbacks.
Copyright (c) 2023 Rifdah Rofifah Zulkifli

This work is licensed under a Creative Commons Attribution 4.0 International License.
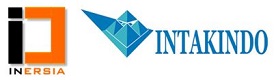
